1.5 billion people around the world do not have access to the services of a bank or similar financial institution. These individuals are referred to as ‘unbanked.’ For the rest of us, less than one-half of the banked population are eligible for lending. To extend the ability of banks to issue loans, smarter credit scoring solutions are evident.
AI-based credit scoring is the most promising and relevant solution. Credit scoring evaluates how well a bank's customer can pay and is willing to pay off debt.
AI-based credit scoring decisions are formed from data such as:
- Total income
- Credit history
- Transaction analysis
- Work experience
- User behavior analytics
Scoring represents a mathematical model based on statistical methods and calculations for a large amount of information.
As a result, AI scoring provides more sensitive, individualized credit score assessments based on an array of additional real-time factors. This allows more people with income potential to access financial services. To gain a deeper understanding of how machine learning is revolutionizing this field, read our detailed exploration in credit scoring using machine learning. This article provides insights into the data-driven nature of AI credit scoring, a cornerstone of modern financial analytics.
Understanding AI Credit Score and Its Meaning
AI credit score, or artificial intelligence credit scoring, is a modern approach to assessing a borrower's creditworthiness. Unlike traditional credit scoring, which relies on static variables and historical data, AI credit scoring uses machine learning algorithms to analyze a wide range of data points, including non-traditional data, to predict a borrower's likelihood of repaying a loan. The AI score meaning, therefore, represents a more comprehensive and dynamic assessment of credit risk, providing lenders with a more accurate and nuanced understanding of a borrower's financial behavior. For further insights into the practical applications and real-world scenarios of AI in credit assessment, our article on AI for credit modelling use cases offers a comprehensive overview.
How Does AI-Based Credit Scoring Work?
AI-based credit scoring revolutionizes the traditional credit assessment process by leveraging advanced machine learning algorithms to analyze a vast array of data points, far beyond what traditional scoring systems consider. This approach enables a more dynamic, comprehensive, and accurate evaluation of a borrower's creditworthiness.
Here's a breakdown of how it works:
Data Collection and Analysis
The first step in AI-based credit scoring is the collection of data. Unlike traditional models that primarily rely on credit history, AI systems can process and analyze a wide range of data sources, including bank transactions, bill payments, social media activity, and even mobile phone usage patterns. This extensive data collection allows for a more holistic view of an individual's financial behavior.
Machine Learning Models
The core of AI-based credit scoring lies in its use of machine learning models. These models are trained on large datasets to identify patterns and correlations that might indicate a borrower's likelihood of repaying a loan. By analyzing thousands of data points, machine learning algorithms can uncover subtle relationships between seemingly unrelated factors and a person's financial reliability.
Predictive Analytics
Once the machine learning model is trained, it can be used for predictive analytics. When a new credit application is received, the AI system evaluates the applicant's data against the patterns learned during training. It then generates a score that predicts the applicant's creditworthiness. This score is based on a complex analysis of various factors, including those that may not be immediately obvious, even to financial experts.
Continuous Learning
One of the key advantages of AI-based credit scoring is its ability to learn and adapt over time. As the system processes more applications and receives feedback on its predictions (e.g., whether borrowers actually repay their loans), it can refine its models to improve accuracy. This continuous learning process ensures that the AI system remains up-to-date with changing financial behaviors and market conditions.
The Role of Artificial Intelligence in Credit Scoring
Artificial intelligence plays a transformative role in credit scoring. Traditional credit scoring models often fail to account for the complexity and variability of individual financial behaviors. AI, on the other hand, can process vast amounts of data, identify patterns, and make predictions with a high degree of accuracy. This allows for a more personalized and fair assessment of creditworthiness. AI credit scoring also has the potential to extend credit opportunities to underserved populations, such as those with thin credit files or those who are new to credit, by considering alternative data in the scoring process.
How AI-based Credit Scoring is Different from Traditional Models
Credit scoring traditionally hinges on the scorecard approach, reflecting the methodologies established at their creation. This conventional system necessitates a substantial history of borrowing behavior for a potential borrower to be deemed "scorable." This requirement often results in a significant barrier for newcomers to the banking sector, who, despite potentially being creditworthy, find themselves ineligible for credit due to a lack of historical financial data.
Moreover, the traditional scorecards have a limited lifetime because the key attributes commonly vary throughout time, so-called population drift. This might be explained by changing economic conditions or new credit strategies (new target audience, new credit products, etc.) In this case, the financial institution risks losing the precision of the credit default estimation resulting in financial loss.
Unlike traditional credit scoring methods (e.g., the scorecard method), by focusing on the past performance of a borrower, AI credit scoring models built with Datrics are more sensitive to real-time indicators of the potential borrower's creditworthiness, such as:
- Current level of income
- Employment opportunities
- Potential ability to earn
Borrowers with high potential (for instance A/B credit score) are invited to participate in the credit programs, while those who formally pass the conventional credit scoring assessment (e.g., credit card churners) are excluded from them.
In other words, AI-based credit scoring allows for more precise profit predictions based on the smart AI models.
Advantages Over Traditional Scoring
AI-based credit scoring offers several advantages over traditional methods:
- Inclusivity: By considering a broader range of data points, AI can provide credit scores for individuals with little to no traditional credit history, thus improving financial inclusion.
- Accuracy: The use of machine learning algorithms allows for more accurate predictions of creditworthiness, reducing the risk of default.
- Efficiency: AI systems can process applications and generate scores much faster than manual methods, speeding up the lending process.
- Adaptability: AI models can quickly adapt to new data and trends, ensuring that credit scoring criteria remain relevant and effective.
Overcoming AI Credit Scoring Challenges: Transparency and Bias Mitigation
AI credit scoring, while revolutionary, is not without its challenges. The main criticisms revolve around the opacity of machine learning models (often referred to as the "black box" problem) and the potential for biased decisions. Datrics has developed solutions to address these issues.

The second figure is the visualization of the features' attributes that force to increase or decrease the prediction. The prediction starts from the base value - the average of all model responses. Each Shapley value is depicted as an arrow that makes the model increase or decrease the prediction.
Tackling the Black Box Problem
A significant concern with machine learning-based credit scoring is the opaque nature of decision-making processes. To combat this, mechanisms for model explainability have been introduced, enhancing transparency on both a general and a case-by-case basis. This approach allows analysts to identify the most influential factors behind any decision, ensuring a clearer understanding of the model's workings.
Credit Default Risk Prediction Interpretation
The use of visual tools has been instrumental in interpreting credit default risk predictions more intuitively. For example, illustrations that show the cumulative impact of various features on the model's output help analysts see how different factors influence predictions. This visualization, often through color-coded dots representing model responses, clarifies the relationship between features and their impact
Visualization of Features' Impact on Predictions
Further demystifying the prediction process, tools that visualize the influence of individual features on predictions have been developed. By starting from a base value and using Shapley values represented as arrows, these tools provide a vivid depiction of how each feature contributes to the final prediction, enhancing the interpretability of the model's output.
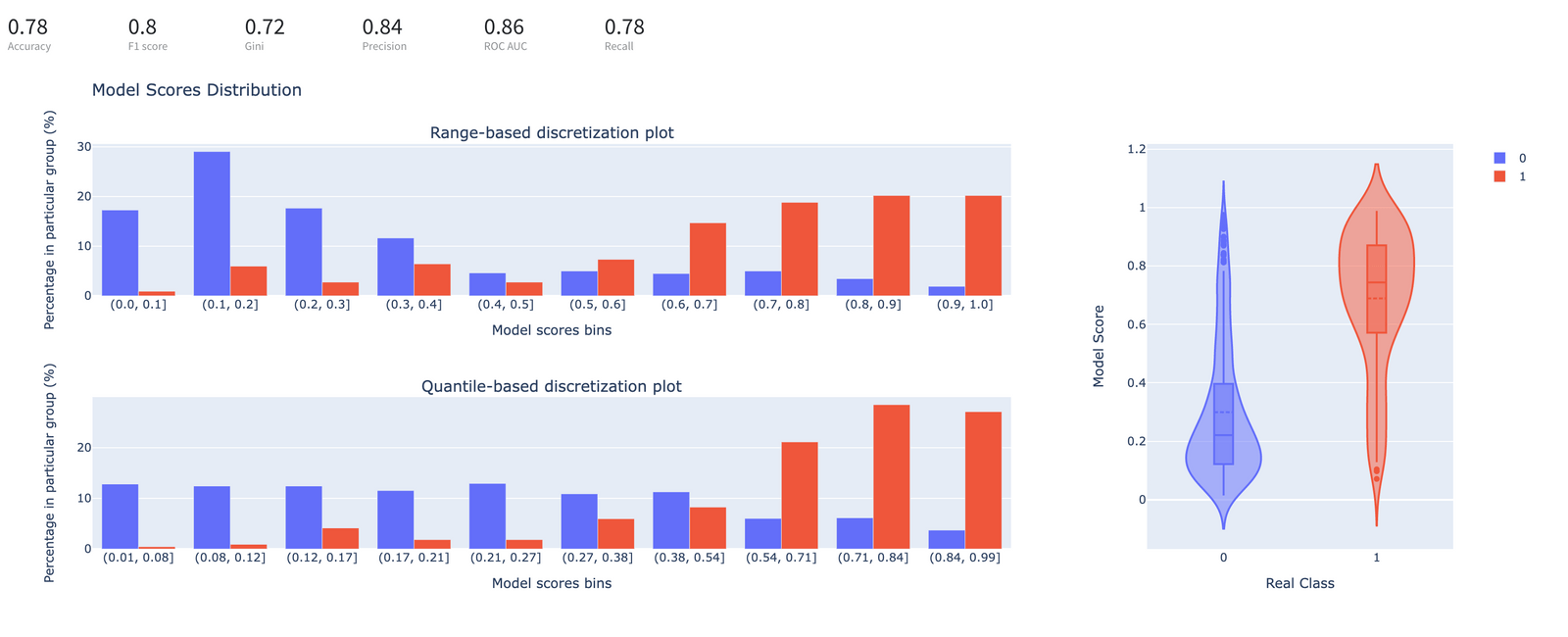

As the model predicts the credit default, the partial scores, which contribute to the credit-worthy score, have the opposite sign to the model coefficients. High partial scores characterize the low-risk groups (A and B) with the risk default probabilities range probabilities less than 15% and 25%, correspondingly. In comparison, the high-risk group (E) relates to a default probability higher than 75%.
Integrating ML with Traditional Scoring for Comprehensive Analysis
A novel approach has been introduced that integrates the advanced capabilities of machine learning with the clarity and familiarity of traditional scoring methods. This hybrid model transforms statistical models into traditional scoring cards, addressing common criticisms of AI-driven credit scoring by combining the best of both worlds.
Detailed Model Score Distribution Visualization
To aid in understanding, a Model Score Distribution plot presents the distribution of output scores across different target classes. This detailed visualization, including elements like the probability density function and discretization plots, allows analysts to observe the distribution of scores and understand the model's predictions better.
Addressing Decision Bias
To tackle the issue of decision bias, a stratified approach to modeling and manual weighting has been implemented. This strategy significantly reduces the risk of bias, ensuring that the scoring model remains fair and does not disproportionately favor or disadvantage any particular group or region.
Credit Scoring Process
The Credit Scoring process consists of the following steps:

Benefits of AI Credit Scoring

Accelerating Decision-Making
AI-enhanced credit scoring models significantly expedite the lending decision process. Unlike traditional methods that rely on decision trees, regression, and complex arithmetic analyses, AI models efficiently handle a mix of redundant, unstructured, and semi-structured data. This capability enables the formulation of more informed, real-time credit decisions.

Promoting Financial Inclusion
AI-driven credit scoring democratizes access to credit, empowering more individuals and businesses to secure funding and bring their ideas to life. The technology simplifies obtaining a first credit report by evaluating financial projections based on income potential and employment opportunities, making credit more accessible to a broader audience.

Streamlined
lending processes
Utilizing AI for credit scoring and lending decisions allows financial institutions to adopt a more data-centric approach. This shift enables lenders to focus on maximizing margins rather than merely minimizing risks, smoothing out the risk vs. profit analysis. As a result, lenders can expand their customer base and enhance profitability, all while making the lending process more efficient.
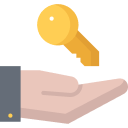
Making AI Accessible to All
The goal of integrating AI into credit scoring is to make this advanced technology available where it's most needed, opening up opportunities for various industries to address financial and social challenges with AI solutions. The design of these AI credit scoring systems emphasizes user-friendliness, clarity, and a no-code approach, ensuring that a wide range of users can easily understand and utilize the models.
Step Into Advanced Credit Risk Management with Datrics
With Datrics, you can build credit scoring to perform precise credit risk assessments and credit scoring based on masses of data, enabling accurate eligibility forecasting and smart borrower ratings. Besides substantial human resource savings, the AI credit scoring systems by Datrics help lenders address "bad" loans via intelligent customer segmentation and forecasting algorithms.
Book a demo with us, and find whether Datrics is the right tool for you.
FAQs
1. How is AI used for credit scoring?
AI is used in credit scoring to analyze vast amounts of data, including non-traditional data sources, to assess a borrower's creditworthiness more accurately and inclusively than traditional methods.
2. Can AI fix my credit score?
AI itself cannot directly fix your credit score, but it can provide more nuanced credit assessments that might favor individuals with non-traditional credit histories, potentially improving access to credit.
3. What is a credit AI score?
A credit AI score is a creditworthiness assessment generated by artificial intelligence algorithms that analyze a wide range of data points, including some that are not considered in traditional credit scoring models, to predict a borrower's likelihood of repaying a loan.
4. What is generative AI for credit scoring?
Generative AI for credit scoring refers to the use of AI models that can generate new data points or simulate scenarios based on existing data to predict creditworthiness, offering innovative ways to assess and manage credit risk.