A planned approach is essential for smooth functioning of any business but especially important for retail and e-commerce industries. You should monitor stock replenishment, ensure regular supplies, analyze the market and trends, predict the sales flow. It's difficult to manage all the stages and succeed in everything.
If you're an E-commerce Executive, Product Owner, Data Analyst, Inventory/Supply Chain manager or Replenishment manager, you have probably faced with the situation of lack or overabundance of items at the storage. At first sight, it's difficult to predict accurately how many goods you will need. Of course, large companies have analytical departments to collect, compare and analyze the data. Smaller businesses usually don't have enough resources. An analysis is undoubtedly an important part of work, but it takes a lot of time to provide precise information. Data science can help with the optimization and automation of analytical routines.
Problems while making a retail business
Retailers are under great pressure as they need to be aware of developments in online ordering and the increasing number of order fulfillment options, from express delivery to curbside pickup. Sometimes there are lots of other challenges and issues in a daily work of e-commerce executives. It depends on the shop's items and specificity, on its size. We defined the most common and most important problems that a person from retail and e-commerce could face:
- Understocking
Understocking is the situation opposite to overstocking, when you provide an insufficient quantity, as of merchandise, supplies, or livestock. It happens when you don't want to overpay for products in order to avoid product spoilage and idle storage. Increasing the out of stock instances leads to possible financial losses. If you have a lack of demanded products, you will likely spend extra money for delivery. Understocking can lead not only to financial difficulties but also can damage the credibility of your company - Overstocking
Overstocking is the situation when a company has more goods or supplies than are needed. Almost every shop or retailer had such an experience. It usually happens when you have a high demand for any item and decide to buy more in order to stay on the safe side. Marketable goods should always be in plenty.
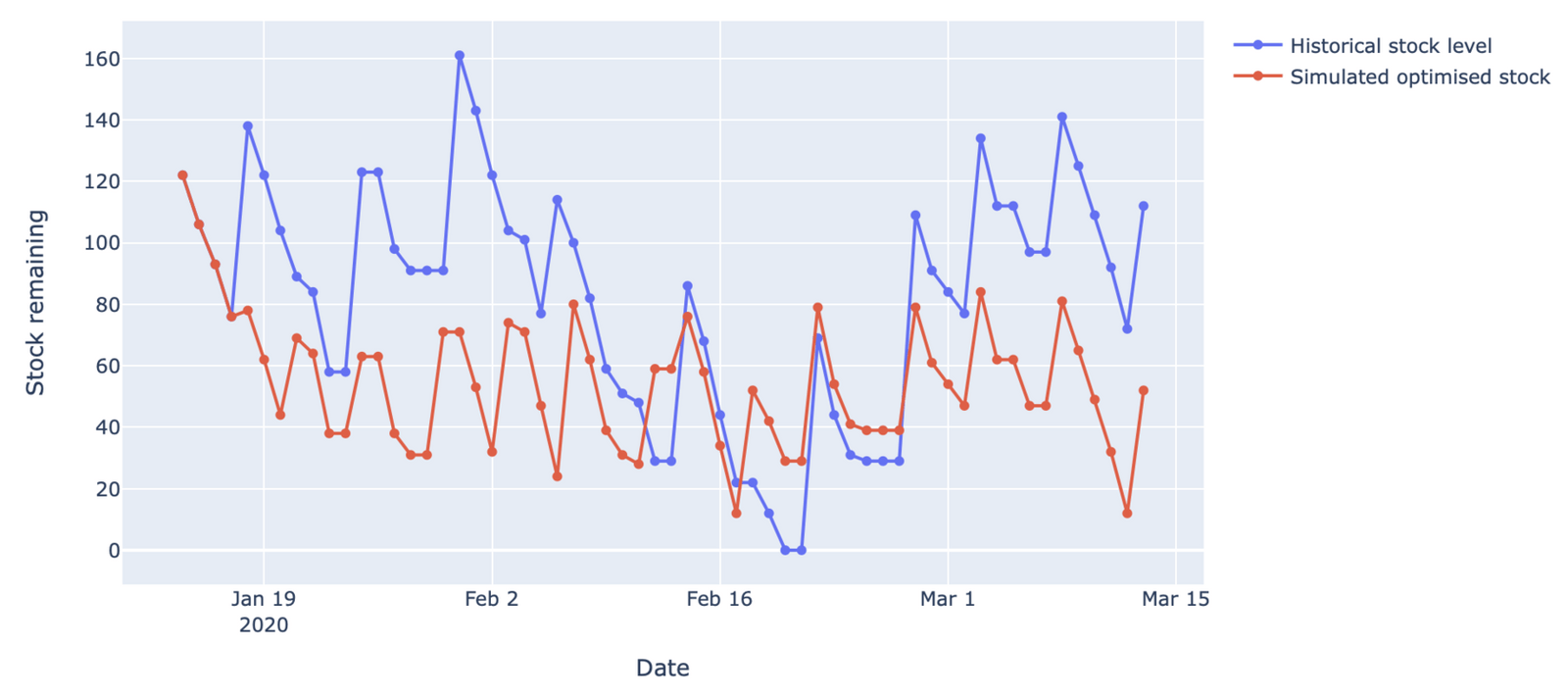
The situation when something is out of stock is an inconvenience both for the customer and shop assistant. It's really important to meet the expectation of clients, but sometimes it leads to overstocking. If you had inaccurate stats or didn't take into account trends and seasonality, your goods could lay around in your pantry or warehouse for years. It could waste your time and a huge amount of money especially if there are lots of such items. The problem of overstocking is most crucial for food production, as oversupply leads to further spoilage.
What is a potential product spoilage?
Of course, potential product spoilage doesn't relate to all goods, it depends on the items you sell. This problem mostly relates to food and flower production. It these fields your schedules should be precise as the price of mistake of retail store management can be too high. To avoid food spoilage companies waste lots of money for express delivery. Online shopping is especially challenging for food retailers as the value of grocery products is too low, in addition it's combined with the high cost of handling products that are often frail, come in variety of sizes and forms, and require temperature-controlled storage and transportation. Currently, only a few large manufacturers are able to make money on e-grocery. Nowadays the emergence of online can't be ignored by food suppliers.
But you're mistaken if you think that other fields of retail couldn't face this problem. Spoilage units are those that do not meet the intended specifications of the customers and are either discarded or sold at reduced prices. It can be defective shoes and clothes as well as defective plastic items that get sold to plastic manufacturers for remelting to produce other plastic products.
Normal and abnormal spoilage should be defined by the business owner according to the company's specificity. On the one hand, we have a normal spoilage index that will be different for the supplier of fruits and vegetables and for cereal manufacturers. This percentage is normally written into the cost of goods sold. On the other hand, we have abnormal spoilage. It extends far beyond the acceptable limit. Such spoilage is always charged as expenses incurred or a separate cost without the ability to be recovered.
Inventory management can normalize indexes of product spoilage and optimize all other processes.
The way of optimization: retail inventory management
We defined the most common problems as overstocking and understocking. One of the most effective approaches is inventory management.
Inventory management is the process of ordering, storing, and using a company's inventory. These include monitoring not only raw materials or components but also finished products, as well as warehousing and processing such items.
An inventory is one of the most valuable assets almost in every company. In retail, manufacturing, food production, and other inventory-intensive sectors, the core of the business is an input of the company and its finished products. A shortage of registered items when and where it's needed can lead to unpredictable consequences. For these reasons, retail inventory management is important for businesses of any size. Knowing when to restock certain items, what amounts to purchase or produce, what price to pay—as well as when to sell and at what price—can easily become complex decisions. Small businesses will often keep track of stock manually and determine the reorder points and quantities using Excel formulas. Larger businesses will use specialized enterprise resource planning (ERP) software. The largest corporations use highly customized software as a service (SaaS) applications.
For better analytics in inventory management can be used demand prediction - a field of predictive analytics that tries to understand and predict customer demand to optimize supply decisions by corporate supply chain and business management. the only thing is needed for a demand prediction is historical data. Based on the information you can have a dimple statistics and understanding of the future flow.
If you sell only one simple item, you probably can do it on your own. For larger companies is completely necessary to have an analytical tool that will combine all the details such as seasonality and trends. Algorithms usually work great with complex data and provide accurate quarterly and yearly predictions.
Minimum needed data for analysis
As we figured out, the most important thing for analysis is data. But what kind of data should it be? In what format? For what period of time?
For deep analysis is usually used a historical data. Historical data includes most data generated either manually or automatically within an enterprise: log files, financial reports, project and product documentation, statistics. Past-periods data used usually as a basis for forecasting future data or trends.
You need at least few months of historical data in CSV format for demand forecasting. It's difficult to get precise analytics with such amount of data, but you can get a preliminary prediction. Few months of historical data will work better for fast-moving goods.
For more accurate analytics you will need a year of available historical data. More information is always better, as software algorithms deal best with a variety of records and simply catch complex patterns.
Data Science solutions for retail/ e-commerce businesses
Nowadays Data science has a huge impact on various industries, especially on retail and e-commerce.
Inventory management could be easily organized and optimized with SaaS. Data analytics helps companies to concentrate on the most valuable goods which are actually the core of the revenue of companies.
Data science software can help with the optimization of goods. In some cases, the velocity of the turnover of goods in warehouses affects companies' revenue. Analytical inventory management tools can reorganize the replenishment process and optimize income.
Simply automation of processes. Manual work and analysis usually waste a lot of time. SaaS platforms resolve it with automation. There is no need for additional work, no coding, no manual efforts. The software just suggests you numbers based on your historical data analytics. All you need is just to make a decision.
Datrics platform as a solution
As we already mentioned, Data science can help with automation and optimization. There is plenty of different software options both for individuals and enterprises. Most of the solutions require additional skills that e-commerce executives may not necessarily have. Datrics affords retail companies the way to leverage their own and public data without writing a single line of code. The platform helps with solving the problem of overstocking and understocking, demand forecasting, pricing analytics.
Advantages:
The platform is fully automated. It doesn't require hours of coding or no manual effort for model training. Datrics builds prediction models automatically, handles pre-processing, actualization, maintenance. You can focus only on decision-making and business strategies.
Time-saving solution: there are no installation or settings processes. You can deal with analysis in a few clicks. All analytical models are already implemented into the platform. All that you need for demand prediction is to upload your pipelines and make a decision about suggested numbers here.