This time we made great improvements to the platform features and functionality such as Big Data support, Binning, MSSQL Connector etc.
Big Data support
Datrics has changed its architecture from Pandas to Dask. Currently computations are available on any datasets with an infinite size.
Parquet
All datasets are automatically converted to parquet format that ensures faster computations.
Model meta-files
Now you can download the file that describes how your model was trained, which steps were performed to convert the initial data to the train dataset. It also describes all the requirements (python libraries) that were used to train the model.
Model performance dashboard
We've updated the model performance dashboard with additional metrics: ROC, AUC, GINI.
Model classification report
Model performance dashboard was extended with the classification dashboard: confusion matrix, detailed classification report, precision/recall and ROC/AUC curves, and a lot more were added.
Binning
We've added the possibility to convert continuous variables to categorical depending on the distribution of the values. Binning functionality supports three auto-binning algorithms - quantile-based, interval-based, and optimal with respect to the Information Value categorization, which is extended with manual adjustment of the bins.
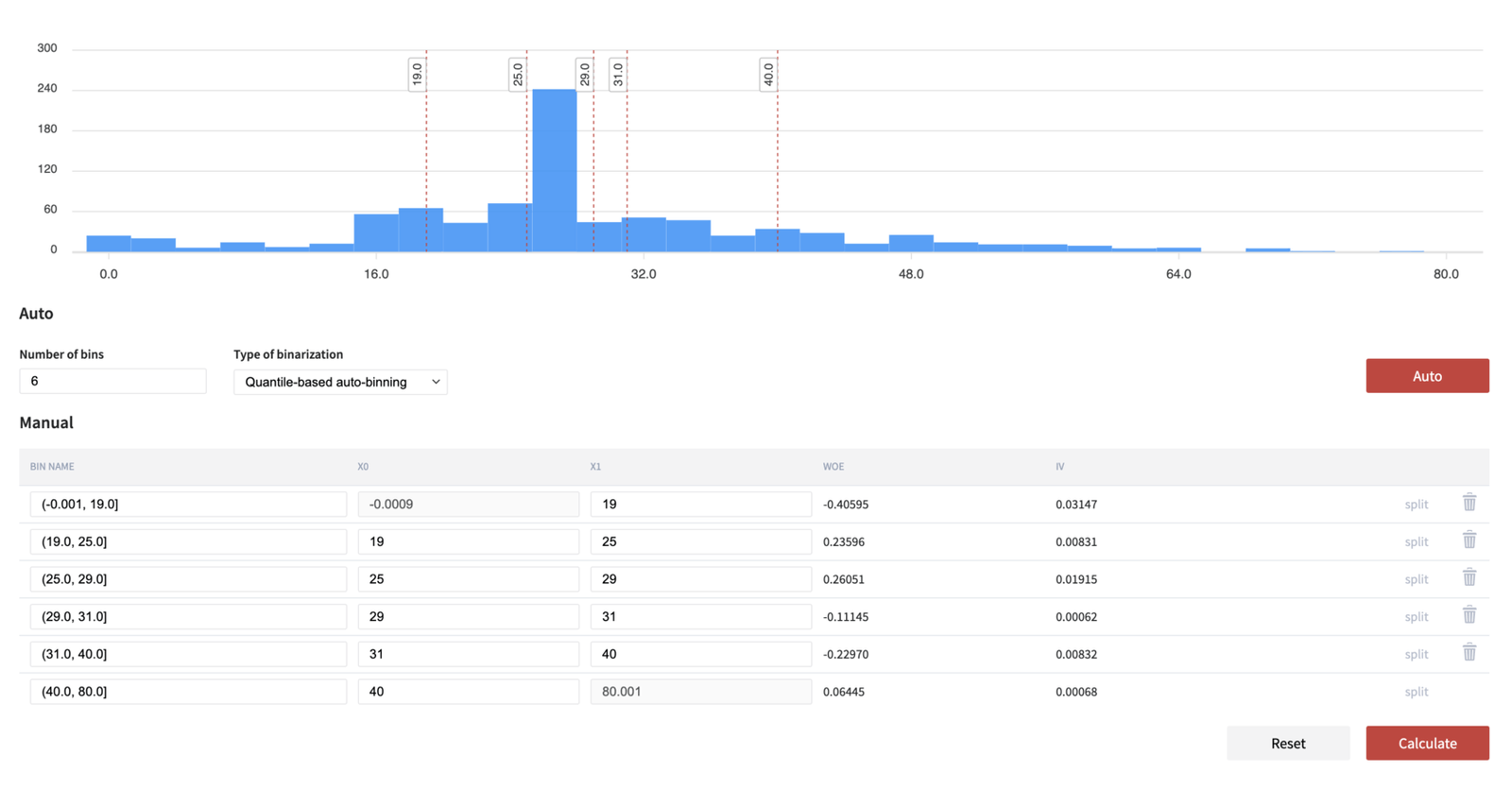
Scorecard
We've added the possibility to take the trained logistic model and calculate the effect of each binary variable to the resulting predicted score. Scorecard functionality supports two types of scores' scaling and allows to perform the adjustment of the scores based on the novel information.
Better What-If API model
Model "What-If API" now supports any size of models, predictions are performed in the dask cluster and support big data sets.
MSSQL Connector
We've added the possibility to extract data from and store data to MS SQL databases.
Variable selection
We've added the possibility to select independent variables that are characterized by sufficient predictive power with respect to the binary target variable.
Information value
We've added the possibility to assess the predictive power of independent variables with respect to the binary dependent variable.
Freeze
For some bricks, the distribution of the training data should be "memorized" for future runs on the unseen data. Freezing capabilities, we've added, allow to store the configuration of the brick and re-use the values for new data.
LogReg
We've added additional binary classification model. This model has additional explainability (e.g. variable coefficients and their statistical significance, etc.) compared to other classification models.
Exploratory Data Analysis
We extended the analytics functionality with Exploratory Data Analysis brick, which provides more insights into analyzed data, like central tendency, dispersion, distribution and outliers.