In today's world, terms like "AI", "Data Science", and "Machine Learning" are everywhere. It seems like every day there is news about ChatGPT's accomplishments, bots beating grandmasters in chess, self-driving cars, or new AI-driven products from Big Tech that make our lives easier. Just a decade ago, all of these things sounded like they belonged only in a science fiction novel or in academia.
The recent surge in interest among organizations is due to the world's shift towards a data-driven economy. Most people have smartphones, computers, or IoT devices, each of which generates hundreds of direct or indirect queries to the web, both sharing its data and gathering new information from the web. Mobile Data Traffic alone produces over 47.6 million terabytes of data per month, which is expected to exponentially increase each year. Google processes 5.6 billion searches every day.
All of this data, whether technical or personal user information, is incredibly valuable to businesses. Companies want to understand their customers: their needs and interests, and how companies can improve their products to serve customers better. However, while companies have access to this data, it is useless in its raw form - what is convenient for machines is rarely understandable to humans. So how can millions of unique transactions be processed in a way that is useful to people?
This is where Data Science comes in to help.
What is Data Science and Analytics?
Understanding Data Science and Its Significance
Data Science is an interdisciplinary field that aims to extract valuable insights and knowledge from data. It combines mathematics practices, such as statistics, probability theory, linear algebra, and calculus, with computational methods to generate intellectual analytics on structured and unstructured data. While simple mathematical operations may suffice for simpler tasks, more complex problems require advanced Data Mining and Machine Learning techniques.
Beyond technical skills, jobs in both data science and data analytics require active communication with clients or stakeholders to understand their needs and acquire domain knowledge.. This expands your knowledge on numerous topics and develops a diverse set of competencies outside the regular technical skills. This part, combined with enigmatic and ingenious algorithms that you learn on your journey, makes data science charming for many specialists.
Data Science helps businesses, organizations, and individuals solve tasks of different natures. For example, it can:
- Help financial institutions calculate default risks and perform risk analysis
- Optimize logistics operations and implement predictive maintenance businesses
- Create a portfolio of trading bots for brokers
- Aid medical specialists in finding cures for diseases
- Get customer analytics for optimizing the marketing efforts
- Allow farmers to control and observe food growth
- Customer 360 Insights, including LTV forecasting
- Demand forecasting, inventory optimization, and revenue forecasting for retailers
The list is extremely large, and every professional can tell you a story of unique projects that data science has made possible.
Analytics: Transforming Data into Actionable Business Intelligence
While Data Science lays the foundation and crafts the toolkit for data understanding, Analytics refines that understanding, turning raw data into actionable intelligence. Analytics delves into past data, spotlighting trends, gauging the impacts of past decisions, and assessing the efficacy of various strategies.
To put it succinctly, while Data Science is the engine propelling our data-driven decisions, Analytics is the compass directing us toward informed conclusions. The true power isn't just in accruing data but in harnessing it for tangible impact.
Organizations turn to analytics for
- Spotting patterns that can predict future trajectories.
- Boosting operational efficiency and trimming costs.
- Crafting strategies that resonate with their target audience.
- Proactively identifying and mitigating risks.
- Grounding decisions in data rather than mere hunches.
In the grand scheme, Data Science is the methodology, and Analytics provides the clarity. Together, they morph data from abstract figures into concrete, actionable narratives.
What is the difference: Data Science vs Artificial Intelligence vs Machine Learning
Data science, AI, and machine learning are often used interchangeably, but they are distinct fields with interconnected components.
- Data science is an interdisciplinary field that uses computational and mathematical methods to extract insights from data.
- AI refers to the ability of machines to perform tasks that typically require human intelligence, such as visual perception, speech recognition, and decision-making.
- ML is a subset of AI that involves training algorithms to make predictions or decisions based on data, and it also adds the possibility for AI to adapt to dynamic environments.
In summary, data science serves as the broader umbrella that encompasses elements of AI and ML. However, not all AI and ML applications fall under the realm of data science. These distinctions are vital in understanding the roles and relationships among these fields. Their relationships can be visualized as follows:

Data Science Practitioners: Roles and Tasks
Data science practitioners are professionals who work in the field of data science. There are several roles within data science and analytics, including data engineer, data scientist, data analyst, and ML engineer. The debate between data engineering vs. data science and machine learning vs. data science often arises due to the distinct tasks associated with each role. Each role has its specific tasks, knowledge requirements, and responsibilities. On a higher layer, these roles can be described as follows:
- Data Engineer: This role is responsible for designing and maintaining the infrastructure required for storing and processing large volumes of data. They optimize databases and data warehouses, build scripts to extract data from various resources, perform data ingestion, and perform data cleaning and data transformation with a goal for the input data to be suitable for future analysis. The required stack of skills includes being able to work with cloud computing, programming, and expertise in algorithm optimization. They rarely utilize any AI tools, but their part in the data science team is immeasurable.
- Data Analyst: the main objective of the data analyst is to perform data analysis to extract insights and knowledge that can be used to make future decisions. Often they perform some parts of the data engineer’s job (like data cleaning), but instead of the infrastructure maintenance, they require skills in data visualization, data mining, and data exploration. Data analysts often have a strong background in business and economics. Sometimes they come from Business Intelligence or at least closely collaborate with them.
- Data Scientist: A Data Scientist is responsible for designing and implementing algorithms that can extract insights and knowledge from data. They work on tasks such as building predictive models, clustering, classification, and natural language processing. Data Scientists often have a strong background in mathematics and statistics.
- ML Engineer: An ML Engineer is responsible for designing and implementing machine learning models that can be used to make predictions or decisions based on data. They work on tasks such as feature engineering, model selection, and hyperparameter tuning. ML Engineers often have a strong background in computer science and programming.
Although there is significant overlap between the roles of data scientists and ML engineers, data scientists typically focus more on analytics and modeling, while ML engineers focus more on implementing, deploying, and supporting those models. When it comes to deployment, these specialists are sometimes referred to as MLOps engineers.
Additionally, ML engineers often collaborate with software developers to assist in integrating ML solutions into applications, whereas data scientists communicate more with clients and BI teams.
It's not uncommon for a data scientist or ML engineer to also possess skills in Data Engineering or Data Analysis. Many specialists are proficient in all of these roles and can act as a “multi-tool” for the entire team. Additionally, these specialists may have sub-specializations based on their domain. For example, one ML engineer may have expertise in computer vision, while another may specialize in natural language processing.
It's worth noting that some companies may combine these roles or have different definitions for them. Often, smaller companies tend to not differentiate between these roles, and only big teams may have narrowly specialized experts. Sometimes, organizations don’t understand these roles at all and force their specialists to solve non-related tasks that require different competencies and skill sets.
Data Analytics vs Data Science: Which Is Right For A Business?
At first glance, data analytics and data science might appear interchangeable. However, understanding their distinctions is crucial for businesses to apply the most suitable tool for specific needs.
Data Analytics primarily focuses on processing historical data to identify trends, analyze the effects of decisions or events, or evaluate performance. If a business's goal is to understand its past performance and make informed decisions for the short term, then data analytics is likely the more appropriate choice. Common tasks include
- Assessment of performance metrics
- Spotting sales trends,
- Analysis of customer behavior
Conversely, Data Science encompasses a broader set of tools and techniques, ranging from data processing to advanced predictive modeling. It proves more suitable for businesses aiming to leverage their data for innovative solutions or long-term strategic decisions. Tasks under this domain might encompass:
- Predictive analytics and forecasting,
- Natural Language Processing for Customer Feedback Analysis
- Advanced data-driven product recommendations
In summary, if a business seeks insights into past trends and metrics, data analytics is the avenue to pursue. For more intricate, forward-looking solutions harnessing a broader spectrum of data, data science holds the key.
Data Science Machine Learning: Tools and Techniques
Machine learning (ML), a pivotal subset of data science, automates analytical model building. Through algorithms, systems are designed to learn from and make decisions based on data. Here's an overview of the tools and techniques prevalent in this realm:
Tools:
- Python: A versatile programming language with libraries, including TensorFlow, Keras, and Scikit-learn, dedicated to ML.
- R: Another commendable language, particularly favored in the statistical domain, that provides ML capabilities.
- Jupyter Notebooks: An open-source tool accommodating live code, equations, and visualizations, among others.
- Tableau: Catering to visual analytics and capable of integrating ML insights.
Techniques:
- Supervised Learning: Using labeled data, algorithms are crafted to predict outcomes. Examples encompass linear regression and support vector machines.
- Unsupervised Learning: In the absence of predefined labels, algorithms classify data. Techniques include clustering and association.
- Reinforcement Learning: Models are trained to learn by interacting with an environment and receiving feedback in the form of rewards or penalties.
- Deep Learning: A subset of ML, this technique utilizes neural networks to analyze various data aspects and is especially effective for image and speech recognition.
The practical applications of these tools and techniques span a broad spectrum. From chatbots capable of understanding and processing natural language to recommendation systems on streaming platforms and predictive maintenance in manufacturing, the fusion of data science and machine learning is reshaping industries and propelling efficiency, innovation, and growth.
What is a Data Science Project?
Though often a data science project is just a part of a larger application, product, or internal business infrastructure, such projects have a full development cycle called “pipelines”. The main object of these pipelines is to perform (semi-)automatized data processing and intellectual data analysis. Typically they follow a similar structure:
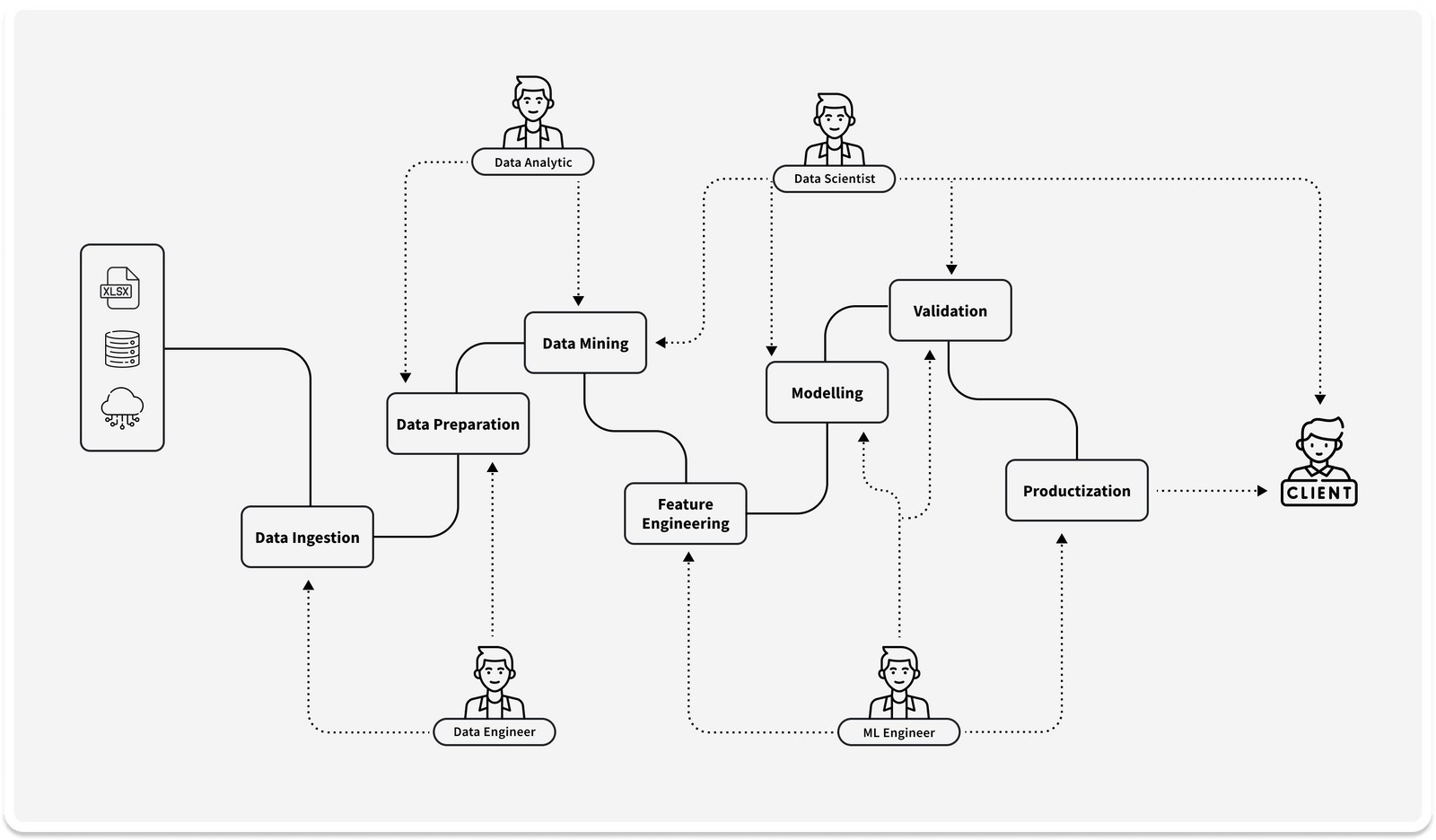
- Data Ingestion: this is a process that involves the collection and import of data from different sources. Among them are databases, data lakes, cloud storage, results of web parsing or API requests, and various structured and unstructured files. This is the direct responsibility of the data engineer
- Data Preparation: often after the ingestion, data is not suitable for further analysis both for humans and machines. This data requires cleaning and transformation (treating missing values, changing data types, scaling numeric values, etc.) to be then further used in data mining. This step is often performed by either a data engineer or a data analyst.
- Data Mining: this step is the first step that implies useful information extraction from the processed data. Data analysts or data scientists try to analyze and determine existing patterns and trends to generate useful insights for further development. Though this step does not always require AI algorithms and is done manually, it is, arguably, the most vital part of the data science project. Data Mining helps specialists to understand the nature of the data, its potential business value, and what tools are needed to solve the task at hand. it also helps us to specify if any additional data is required and if the AI is even needed.
- Feature Engineering: the main task of Feature Engineering is to select, extract, and transform raw input data into useful features that can be used to train machine learning models. The goal of this is to determine important features (like age, salary, color, and form of the visual object) and filter out useless ones. If needed, the features can be artificially created from existing data, and polished by scaling, normalization, encoding, etc. By performing all these actions, ML engineers and data scientists improve ML algorithms’ efficiency to learn patterns in data and make accurate predictions.
- Modeling: this step includes a selection of the most efficient ML model for the task at hand and then training it. While the selection of the model is an important task that requires good qualifications, it is highly dependent on previous steps of the pipeline: even the best state-of-the-art model will fail if data makes no sense. Also, complicated problems, large data volumes, and complex models require significant computational powers to perform this task. While simple models and small datasets can train in a few minutes on a regular laptop, tasks in computer vision and natural language processing may require hours or even days on powerful GPU-based workstations. This step is performed by ML engineers and/or data scientists
- Validation: this step is likely the shortest, though not always the easiest. After the model is trained, we need to check if it truly produces good results on previously unseen data. Sometimes, the model can show good accuracy on train data, but fail on test sets or, worse, during production. After determining that model is not efficient, the next step is to clarify why. Often you might return to previous steps to solve identified problems (also, you might require to return to any step of the pipeline). This process is also performed either by an ML engineer or a data scientist
- Productization: the last step of the pipeline. Once the model is validated to solve the task at hand, we need to integrate the pipeline/model into a larger system or an application to be accessible and usable by end users. This involves deploying the model to a cloud server, integrating it into a software or hardware application, creating the API, and/or creating a monitoring system to track the performance of the model in the real world to adapt/retrain the model if performance is dropped. This step is governed by ML engineers (or MLOps) who may require help from software development teams.
Each of these steps is important, but different projects may not need some of them or may even require additional ones. Some of these steps may be done in parallel, in a different order, or by different roles - sometimes even by a single person. Furthermore, outside of these “pipelines,” the need to communicate with clients, BI and product teams, and other business or development-related tasks still exists.
Summary
Data Science is a wonderful and exciting field of knowledge, it combines both the beauty and usefulness of mathematics, and while being a very young discipline, it already changed our lives and opened new horizons for businesses and individuals. We discussed what data science is, and how it is connected to other enigmatic technologies like artificial intelligence, and covered on a higher level the differences among various data science-related roles. In addition, we went through the basic principles of data science project development and the key steps of the data science pipelines.
Our goal in writing this article was to cover the basics of data science projects and roles so that you could better understand the opportunities data science opens for people and companies in a data-driven economy. At Datrics, we aim to democratize data science, so that more people could utilize AI and ML to achieve their goals.
If you feel you are lacking knowledge in statistics, probability theory, ML technics, etc, don't be discouraged. Most data science practitioners do not require an academic level of understanding, and beginners can tackle difficult tasks with just conceptual knowledge. Similarly, while basic software development skills are essential, Python provides all state-of-the-art techniques in its popular libraries. Additionally, there is a growing number of low-code and no-code solutions that are useful for both beginners and established professionals.
This article is the beginning of the sequence of articles on data science basics where we will dwell deeper into each point made today. We will start with the most intriguing part - machine learning algorithms, where we plan to cover different approaches ML has to solve tasks of various natures and how you can train your own models to solve data-related problems. Stay tuned for more from data science experts from Datrics.